As the digital world grew, design systems became essential allies, bringing consistency and scalability. They helped streamline workflows, unify experiences, and accelerate delivery. Design systems have been transformative in many ways, and now they’re ready for their next evolution.
This is where AI enters the picture, and we’ve worked to bring some really compelling ideas to life.
AI proves especially useful when it comes to handling repetitive, systematic tasks. Designers and developers often spend considerable time on them—time that could be redirected toward solving deeper problems or creating solutions that advance the digital experience industry.
With this in mind, we saw an opportunity to integrate AI into design systems. Our work on assisted solutions over the past year has shown great potential to solve real problems.
By speeding up execution and introducing more systematic processes, we wanted to open the door to:
- Identifying problems correctly and dedicating adequate time to solutions
- Diving deeper into user research and insights
- Prototyping bold, innovative solutions
- Exploring new design directions
- Engaging in meaningful discussions about the impact of digital solutions
- Focusing on strategic decisions that drive measurable outcomes
This blog focuses on the answers we found, solutions that have the potential, and the journey we believe opens exciting possibilities for the industry, as well as for our existing and future clients.
Problems we wanted (want) to solve
Design systems are never one-size-fits-all—they’re built to address the specific needs of each digital platform. This advantage to customization brings its own challenges, and we noticed several recurring ones that could become key use cases for us and AI to address.
- Integrating unique client requirements into a cohesive design system often becomes a challenge. Components don’t always fit seamlessly within the system’s structure, especially when dealing with varied requirements across platforms. Creating custom solutions from scratch requires significant time and effort, often extending project timelines.
- Tech teams spend countless hours aligning new components with the broader design language, frequently repeating similar tasks across projects. While this process is critical for maintaining brand consistency, it reduces the time available for tasks like refining user interfaces for accessibility or conducting usability testing to enhance the overall experience.
- Crafting bespoke solutions tailored to specific industries and clients is a priority. Resistance to change, varying levels of understanding among stakeholders, and differing priorities often slow the process. We aimed to exceed expectations and AI became central to helping us achieve this.
- Many brands operate across multiple platforms, and like mentioned earlier, components that work well on one platform don’t always translate seamlessly to another. This often requires adjustments or the creation of entirely new components, further stretching timelines.
- Collaboration between design and development teams frequently involves extensive back-and-forth to refine components. Even minor updates can take considerable time due to prolonged feedback loops and revision cycles, delaying progress.
- A critical area we also wanted to address was the ability to quickly convert ideas into quick components. This wasn’t a problem but a goal that was destined to help teams validate functionality and compatibility early in the process.
We established that saving time, improving execution, supporting teams, and exceeding expectations are central to our journey. These factors highlighted the need to address challenges like repetitive, systematic processes that consume valuable resources. Recognizing this, we turned to AI to provide practical solutions tailored to these specific needs.
The solutions
1. Intelligent component generation
So, we had to start somewhere. The first solution we tried with AI integration into the design system is component generation—and it’s definitely intelligent. By analyzing prompts, the AI generates components that align perfectly with the existing design language, including colors, typography, and branding.
For instance, a developer might say, “Create a card with an image, title, and description,” and the AI delivers a ready-to-use component. This eliminates the need to manually design or code components, saving time and speeding up workflows.
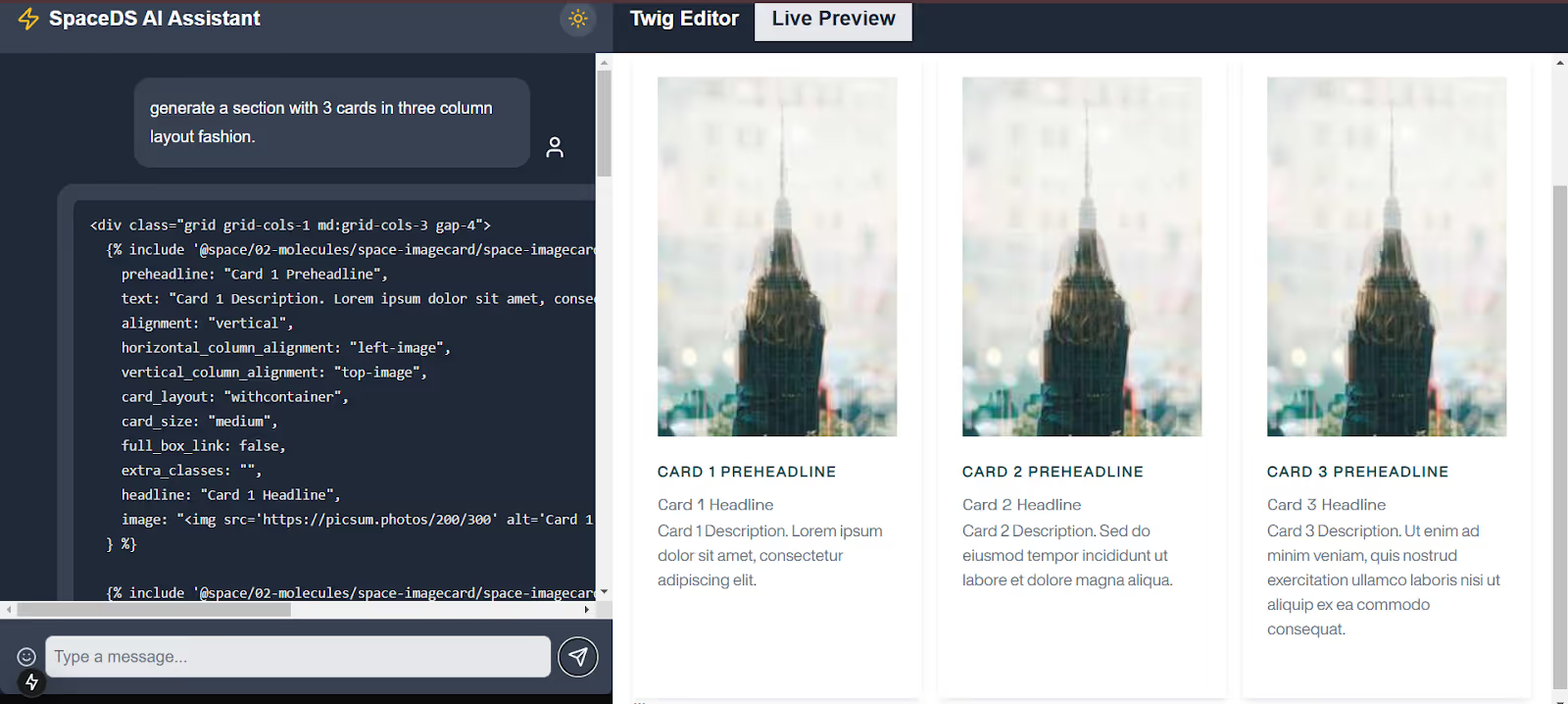
AI-assisted design with live previews for smooth execution
2. Real-time customization
Next, we looked at making changes effortlessly with real-time interactivity. Developers can simply request changes like, “Add a CTA banner” or “Include testimonials,” and the AI makes those updates instantly. This conversational approach cuts out the usual back-and-forth, making customizations quicker and far more agile.
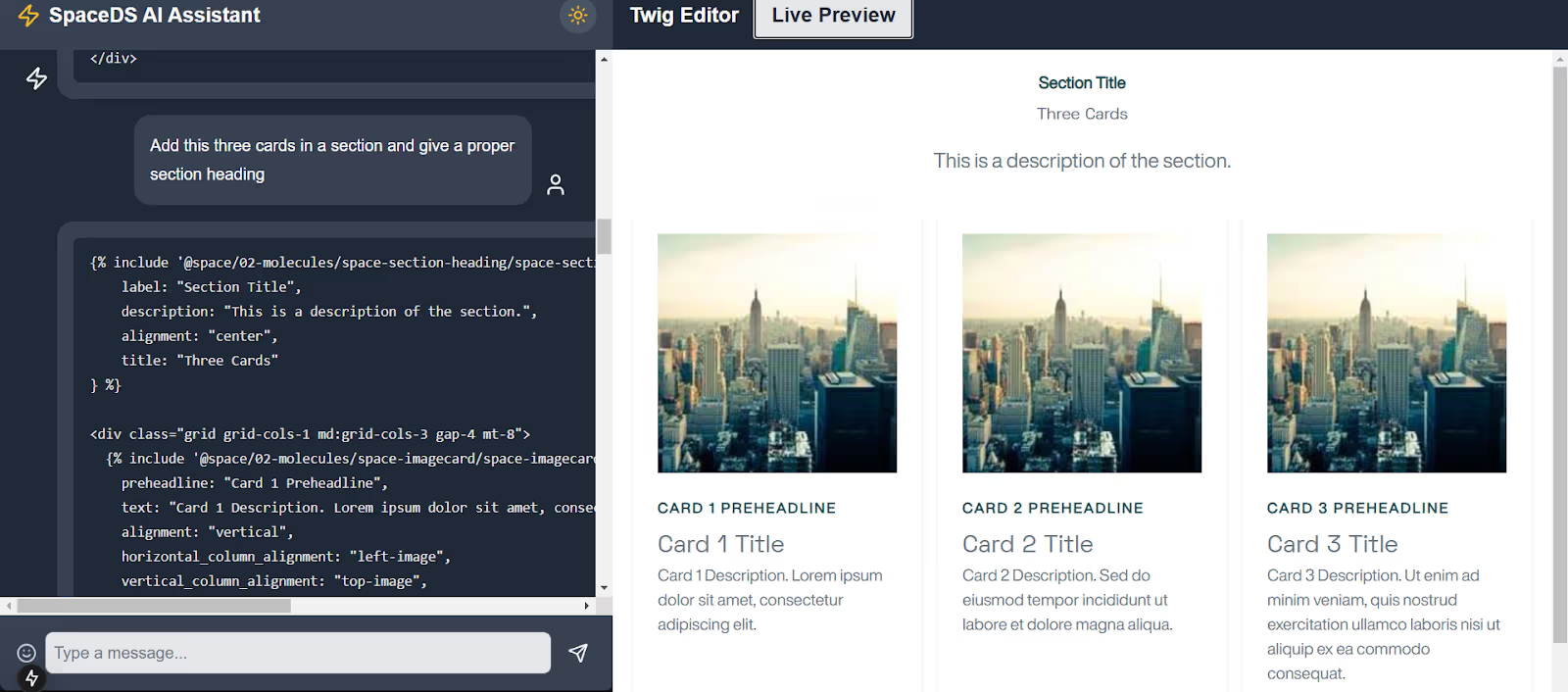
Quickly adjust sections and cards for precise designs
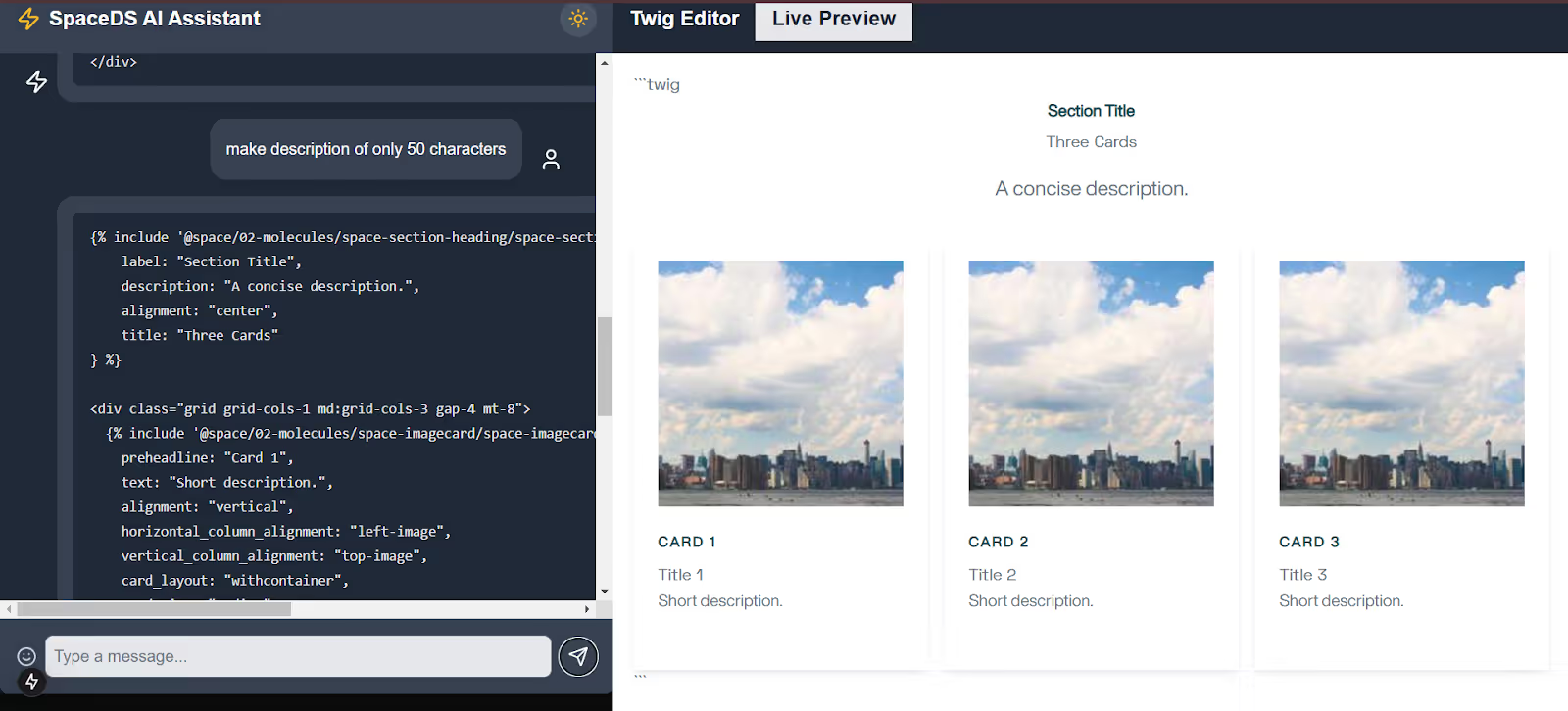
AI customizes frameworks to create unique design components
3. Cross-system flexibility
One of the most valuable aspects of this solution is its adaptability. It works equally well for refining an established framework like SPACE or creating a brand-new design system. Developers define the basics—like buttons, input fields, and headings—and the AI uses these building blocks to craft higher-order components tailored to the project’s specific requirements.

If a component is missing, AI uses atoms and molecules to build a new one
4. Modular agent workflows
When projects get complex, having a structured workflow makes all the difference. With an agent-based system, tasks are broken into manageable parts. For instance, if a client needs a landing page, one agent identifies the key elements—like hero banners, menus, and testimonials—while another assembles these components using the design system. This modular approach keeps everything consistent and speeds up execution.
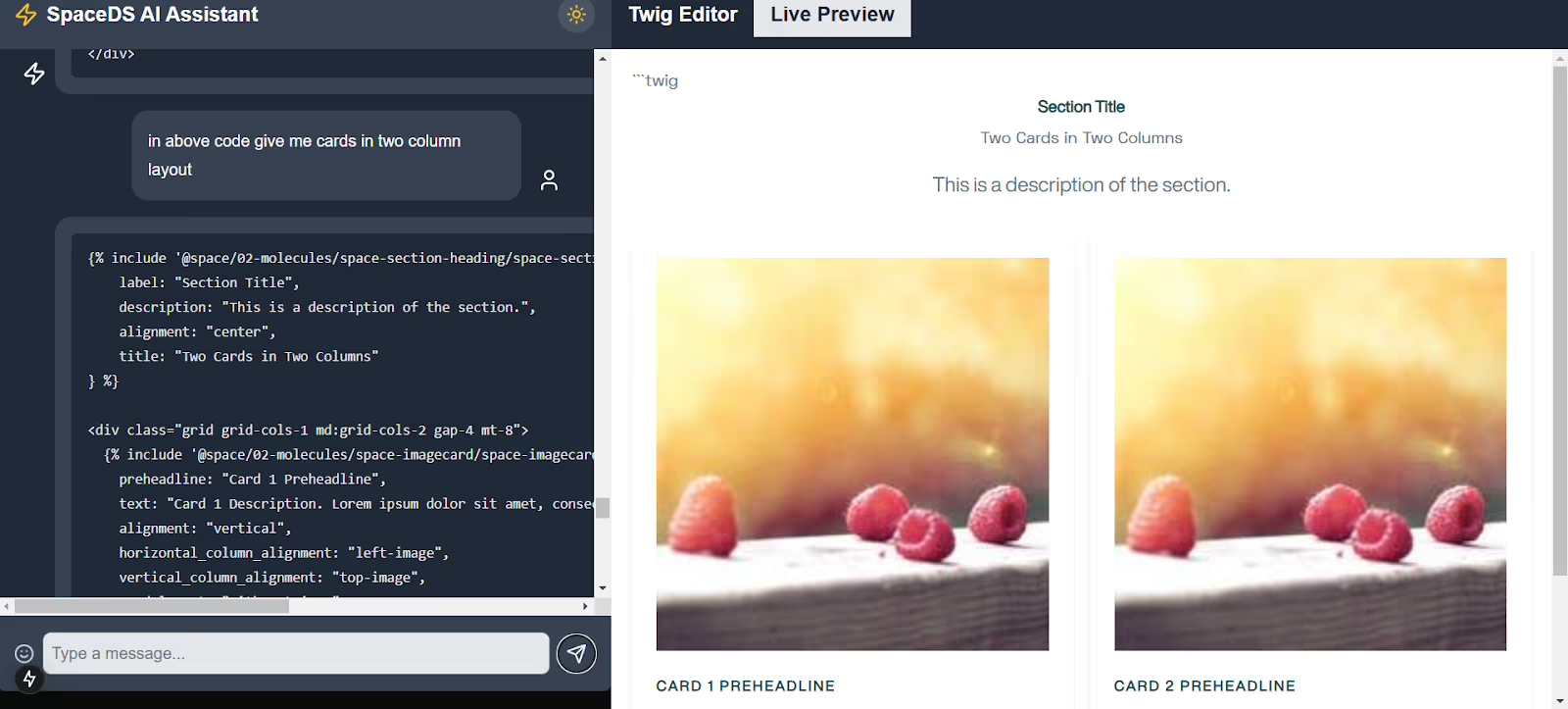
Instant AI updates speed up design iterations
5. Visual Inputs and Cues
As we continue working on AI-assisted solutions for design systems, the next step is integrating visual inputs directly into the workflow. Teams will soon be able to upload Figma screenshots, enabling the AI to analyze elements like buttons, cards, and forms, along with their layout and hierarchy. These visual cues will allow the AI to generate components directly from designs, bridging the gap between static visuals and functional systems. This approach aims to streamline the transition from design to development, ensuring efficiency and clarity across projects.
Well, this is just the beginning of how AI can enhance design systems. While it already supports prompt-based workflows, the next step focuses on integrating components more effectively. As the solution evolves, the priority remains to help teams create components efficiently while staying aligned with the principles of a design system and project goals.
Yes, it is about saving time and speeding up execution, but with the intention—to build a brand that resonates rather than just completing tasks. For designers, this means moving beyond repetitive workflows and focusing on truly understanding users. It’s about addressing business challenges, crafting experiences that add value, and ensuring every decision serves a clear purpose.
For developers, the shift creates space to improve functionality and create adaptable systems that grow with the brand’s needs. By letting AI handle routine execution, teams can channel their energy into creating digital experiences that solve real-world problems and leave a lasting impact.
What we kept in mind while executing new AI-assisted workflows
When integrating AI into design workflows, we focused on ensuring scalability, sustainability, and meaningful outcomes. Each step of the process was guided by key principles that helped shape our approach:
First, we looked at repetitive tasks that take time but don’t directly contribute to strategic goals. Automating these tasks was about shifting the focus to work that drives real impact and value.
We also knew that data readiness was essential. For AI to work effectively, the data infrastructure had to be clean, well-structured, and accessible. Without this foundation, even the best AI systems can fail to deliver consistent results.
Scalability was another major factor. We wanted solutions that could adapt easily across different projects, design systems, and workflows without requiring constant rework. Flexibility was key to ensuring the systems would continue delivering value as needs evolved.
Starting small and scaling thoughtfully was a priority as well. We tested manageable use cases first, refining the system based on real-world feedback. This approach ensured the workflows we built were practical, reduced risks, and evolved to meet real-world needs effectively.
Striking a balance between automation and creativity was equally important. Automation allowed us to address inefficiencies and speed up execution while leaving space for human ingenuity to ensure the outcomes were functional and user-centered.
Vision for what’s next
We are not just looking ahead at saving time (well, not only) — we are focused on ensuring the time saved is used for important, impact-focused work as well. That’s a goal we discuss often.
By automating repetitive tasks, we aim to create systems that allow teams to dedicate their efforts to meaningful, high-value work.
And, the journey doesn’t end here.
The solutions we’ve developed so far are part of an ongoing process of learning and refinement, with each step bringing us closer to building design systems that adapt and scale effortlessly. As we look ahead, our focus remains on aligning AI solutions with human creativity and purpose.
This vision also involves building stronger client relationships by ensuring AI’s role is transparent and its processes clear. When AI’s contributions are easy to understand and align with the project’s goals, it strengthens trust and collaboration between teams and clients, making every step of the process smoother and more productive.
In our opinion, AI-assisted solutions are more than a feature—they are collaborators, agents or could be addressed as assistants in some scenarios. We want to keep working better with this assistant, ensuring humans remain in the loop, at every stage of the processes, contributing to ideas, decisions, and purpose.